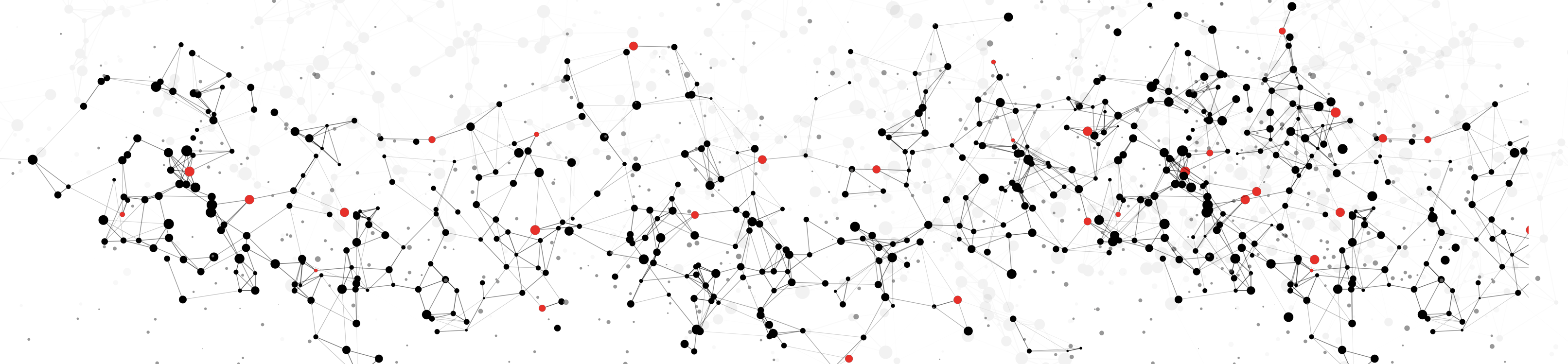
# About event
The ML in PL Conference is an event focused on the best of Machine Learning both in academia and in business. This year, after two years of remote events, we've decided to organize a on-site event with free live-stream for the first time.
Learn from the top world experts
Share your knowledge
Meet the ML community
Feel the unique atmosphere
Watch online for free
# Invited Speakers
-
Petar Veličković
Petar Veličković
Petar Veličković is a Staff Research Scientist at DeepMind, Affiliated Lecturer at the University of Cambridge, and an Associate of Clare Hall, Cambridge. He holds a PhD in Computer Science from the University of Cambridge (Trinity College), obtained under the supervision of Pietro Liò. His research concerns geometric deep learning—devising neural network architectures that respect the invariances and symmetries in data (a topic I’ve co-written a proto-book about). For his contributions, he is recognised as an ELLIS Scholar in the Geometric Deep Learning Program. Particularly, his focus is graph representation learning and its applications in algorithmic reasoning (featured in VentureBeat). He is the first author of Graph Attention Networks—a popular convolutional layer for graphs—and Deep Graph Infomax—a popular self-supervised learning pipeline for graphs (featured in ZDNet). Petar’s research has been used in substantially improving travel-time predictions in Google Maps (featured in the CNBC, Endgadget, VentureBeat, CNET, the Verge and ZDNet), and guiding intuition of mathematicians towards new top-tier theorems and conjectures (featured in Nature, Science, Quanta Magazine, New Scientist, The Independent, Sky News, The Sunday Times, la Repubblica and The Conversation).
-
Matthias Bethge
Matthias Bethge
Matthias Bethge is a Professor for Computational Neuroscience and Machine Learning at the University of Tübingen and director of the Tübingen AI Center, a joint center between Tübingen University and MPI for Intelligent Systems that is part of the German AI strategy. He is also co-initiator of the European ELLIS initiative and co-founder of Deepart UG, and Layer7 AI GmbH.
-
Farah Shamout
Farah Shamout
Farah Shamout is an Assistant Professor of Computer Engineering at NYU Abu Dhabi, where she leads the Clinical Artificial Intelligence Lab. She is also an Associated Faculty at the NYU Tandon School of Engineering (Computer Science & Engineering and Biomedical Engineering Departments) and an Affiliated Faculty at NYU Langone Health (Radiology). Before pursuing the tenure-track position, Dr. Shamout spent three years at NYU as an Assistant Professor Emerging Scholar, during which she received the Campus Life Faculty Leadership Award in 2021. At the Clinical AI Lab, Dr. Shamout is interested in developing machine learning methods and systems using heterogeneous real-world data for applications in computational precision health. This includes electronic health records data and medical imaging for medical diagnostics and prognostics. Methodologies of interest pertain to multi-modal fusion, representation learning, and augmenting machine and human decision-making, to achieve high performance and utility in clinical practice. Dr. Shamout completed her DPhil (PhD) in Engineering Science at the University of Oxford as a Rhodes Scholar and was a member of Balliol College. Her doctoral research focused on developing early warning models using electronic health records data to predict in-hospital clinical deterioration. While at Oxford, Shamout taught with the inaugural UAE-Oxford Artificial Intelligence Program and worked on global data commons and digital health policy. She also completed her BSc in Computer Engineering (cum laude) at NYU Abu Dhabi
-
Piotr Miłoś
Piotr Miłoś
Piotr Miłoś is a professor at the Polish Academy of Sciences and a research team leader at IDEAS NCBR. He specializes in machine learning and has a strong background in probability theory. He is a leader of a research group focusing on a range of topics related to sequential decision-making. The group develops new methods for domains that require reasoning (e.g., theorem proving). These include new planning algorithms and deep learning techniques for sequential modeling. Another principal line of the group's research is continual learning, focusing on reinforcement learning settings. Prof Milos actively works toward developing a machine learning community in Poland. This includes hosting a reinforcement learning seminar and co-organize a reinforcement learning course (first in Poland).
-
Cheng Zhang
Cheng Zhang
Cheng Zhang is a Principal Researcher, leading causal AI for decision making at Microsoft Research Cambridge (MSRC), UK. She is an expert in deep generative models, causal discovery, causal inference, and decision-making under uncertainty. She has published in all top venues in machine learning, including NeurIPS, ICML, AIStats, UAI, and AAAI. Apart from research expertise, she is also experienced in enabling real-world impact in different domains.
-
Andriy Mnih
Andriy Mnih
Andriy Mnih is a research scientist at DeepMind, specializing in machine learning. His primary research interests are generative modelling, variational inference, representation learning, and gradient estimation in stochastic computation graphs. He obtained his PhD on learning representations for discrete data from the University of Toronto in 2010, where he was advised by Geoffrey Hinton. Before joining DeepMind in 2013, Andriy was a post-doctoral researcher at the Gatsby Unit, University College London, working with Yee Whye Teh.
-
Aleksander Mądry
Aleksander Mądry
Aleksander Mądry is the Cadence Design Systems Professor of Computing at MIT, leads the MIT Center for Deployable Machine Learning as well as is a faculty co-lead for the MIT AI Policy Forum. His research interests span algorithms, continuous optimization, and understanding machine learning from a robustness and deployability perspectives. Aleksander's work has been recognized with a number of awards, including an NSF CAREER Award, an Alfred P. Sloan Research Fellowship, an ACM Doctoral Dissertation Award Honorable Mention, and Presburger Award. He received his PhD from MIT in 2011 and, prior to joining the MIT faculty, he spent time at Microsoft Research New England and on the faculty of EPFL.
-
Marc Deisenroth
Marc Deisenroth
Professor Marc Deisenroth is the DeepMind Chair of Machine Learning and Artificial Intelligence at University College London. His research focuses on data-efficient machine learning, probabilistic modeling and autonomous decision making. Marc Deisenroth was Program Chair of EWRL 2012, Workshops Chair of RSS 2013, EXPOChair at ICML 2020, Tutorials Chair at NeurIPS 2021, and Program Chairat ICLR 2022. He received Paper Awards at ICRA 2014, ICCAS 2016, ICML2020, and AISTATS 2021. In 2019, Marc co-organized the Machine Learning Summer School in London. In 2018, Marc received The President’s Award for Outstanding Early Career Researcher at Imperial College. He is a recipient of a Google Faculty Research Award and a Microsoft PhD Grant. In 2018, Marc Deisenroth spent four months at the African Institute for Mathematical Sciences (Rwanda), where he taught a course on Foundations of Machine Learning as part of the African Masters in Machine Intelligence. He is co-author of the book Mathematics for Machine Learning, published by Cambridge University Press.
-
Kyunghyun Cho
Kyunghyun Cho
Kyunghyun Cho is an associate professor of computer science and data science at New York University and CIFAR Fellow of Learning in Machines & Brains. He is also a senior director of frontier research at the Prescient Design team within Genentech Research & Early Development (gRED). He was a research scientist at Facebook AI Research from June 2017 to May 2020 and a postdoctoral fellow at University of Montreal until Summer 2015 under the supervision of Prof. Yoshua Bengio, after receiving PhD and MSc degrees from Aalto University April 2011 and April 2014, respectively, under the supervision of Prof. Juha Karhunen, Dr. Tapani Raiko and Dr. Alexander Ilin. He received an honour of being a recipient of the Samsung Ho-Am Prize in Engineering in 2021. He tries his best to find a balance among machine learning, natural language processing, and life, but almost always fails to do so.
# Panels and Panelists
-
Popularization of ML Research
Piotr is a data scientist with a background in quantum physics. He co-founded Quantum Flytrap, a startup developing a simple UI for quantum computing. He received his PhD in quantum optics theory from ICFO Castelldefels in 2014 and directly moved to the industry. Piotr's experience includes data viz for biotech, lecturing at Imperial College London, delivering trainings at deepsense.ai, and deep learning consulting for clients such as Intel and Samsung. He authored popular blog post introductions to data science and deep learning. His model training visualization library, livelossplot, has over 1M Downloads.
Yannic runs the world’s largest YouTube channel dedicated to Machine Learning Research. His video topics range from technical analysis of new papers to covering the ML community’s recent news and developments, as well as mini-research projects. He holds a PhD in ML from ETH Zurich and is a co-founder of the Swiss LegalTech startup DeepJudge.
After having studied Physics and Computer Science, Letitia is a PhD candidate at Heidelberg University. Her research focuses on vision and language integration in multimodal machine learning. Her side-project revolves around the "AI Coffee Break with Letitia" YouTube channel, where the animated Ms. Coffee Bean explains and visualises concepts from the latest Natural Language Processing, Computer Vision and Multimodal research.
-
Women in ML in PL
Farah Shamout is an Assistant Professor in Computer Engineering at NYU Abu Dhabi, where she leads the Clinical Artificial Intelligence Laboratory, and an Affiliated Faculty at NYU Radiology, New York. Her research interests lie in developing machine learning and data science approaches to tackle real-world clinical problems. Farah completed her DPhil (PhD) in Engineering Science at the University of Oxford as a Rhodes Scholar and was a member of Balliol College. Prior to her doctoral studies, she completed her BSc in Computer Engineering (cum laude) at NYU Abu Dhabi.
Inez Okulska is an Assistant Professor and the Head of the Linguistic Engineering and Text Analysis Department at NASK National Research Institute; a proud co-author of the open-source library StyloMetrix, a grammar-oriented interpretable text analysis tool for English and Polish. In 2022 she was selected as one of the Top 100 Women in AI in Poland. Her career path sets an example for young women to take courage and start in AI, even when originally coming from humanities. She graduated from Cultural Studies, Linguistics, and Polish philology and did her Ph.D. in translation studies. Then, in her 30ies, she enrolled again at the University, this time the Warsaw University of Technology, and started a master's degree in Robotics and Automation. 2020 she graduated with MSc, and her master's thesis was awarded a distinction in the IEEE contest for best master's thesis in Automation.
Agnieszka Dobrowolska is a Machine Learning Scientist at Relation Therapeutics, working on representation learning for biological sequences. Previously, she worked on privacy-preserving approaches for training automatic speech recognition models at Samsung Research. She completed her MSc in Machine Learning at UCL, during which she wrote her thesis on learning new concepts in knowledge graphs. During her BSc in Natural Sciences, she majored in Physical Chemistry with Mathematics and Statistics, focusing on computational chemistry for drug discovery.
Daria Szmurlo is a Senior Principal Data Scientist at QuantumBlack, AI by McKinsey, with 10+ years of professional experience in retail, insurance, healthcare and life sciences. In QB, she leads the development of AI engine for retail, advises companies worldwide on the effective use of data and AI, and co-leads QB in Central Europe. Her background is in mathematics (Jagiellonian University, Cracow), and economic modelling (University of Economics, Cracow). Outside of work, her creative passion translates into various forms of handicrafts, eg getting lost gluing paper while listening to favorite podcasts.
-
Industry and Academia
Przemysław Biecek is a professor at the Warsaw University of Technology and the University of Warsaw. He develops tools, methods and processes to explain predictive models DrWhy.AI. Established a research team MI2.AI that runs a wide range of projects from developing and auditing models used in medical and financial applications, to organising training courses and workshops on DataVis and XAI topics. Author of more than 100 scientific articles, five monographs on data analysis, data visualisation and programming. In his spare time, he is an enthusiast of so-called data-literacy, and is the author of popular science books in the Beta and Bit series.
As Chief Technology Officer, Robert Bogucki has built and currently oversees deepsense.ai’s technical team. Together with the team, he focuses on bridging the gap between AI research and real-life business challenges. In addition to working with clients, Robert also leads deepsense.ai’s research and development team, managing and supporting their efforts in deep learning and reinforcement learning. Apart from his business activities, Robert is also a university lecturer on machine learning and an award winning Kaggle competitor. Prior to establishing deepsense.ai, he gained experience at UBS and co-founded CodiLime.
Pablo Ribalta is a deep learning algorithms manager at NVIDIA Warsaw. Holds a PhD in machine learning and artificial intelligence by the Silesian University of Technology, and has an extensive background in academia and engineering. At NVIDIA, his group drives cutting-edge research in fields like vision, computer graphics and drug discovery, running on state-of-the-art NVIDIA platforms and next-gen NVIDIA GPUs. His interests are connected with state-of-the-art deep learning methods and workloads at scale.
Piotr Sankowski is a professor at the Institute of Informatics, University of Warsaw, where he received his habilitation in 2009 and where he received a doctorate in computer science in 2005. His research interest focuses on practical application of algorithms, ranging from economic applications, through learning data structures, to parallel algorithms for data science. In 2009, Piotr Sankowski received also a doctorate in physics in the field of solid state theory at the Polish Academy of Sciences. In 2010 he received ERC Starting Independent Researcher Grant, in 2015 ERC Proof of Concept Grant, and in 2017 ERC Consolidator Grant. He is the president of IDEAS NCBR – a research and development center operating in the field of artificial intelligence and digital economy. Piotr Sankowski is also a co-founder of the spin-off company MIM Solutions.
Stanislaw Jastrzebski serves as the CTO and Chief Scientist at Molecule.one, a startup solving the chemistry bottleneck in drug discovery. He gained industrial experience at Google, Microsoft and Palantir. At heart both an entrepreneur and scientist. He is passionate about improving the fundamental aspects of deep learning and applying it to automate scientific discovery. He completed his postdoctoral training at New York University in deep learning. His PhD thesis was based on work on foundations of deep learning done during research visits at MILA (with Yoshua Bengio) and the University of Edinburgh (with Amos Storkey). He received his PhD from Jagiellonian University, advised by Jacek Tabor. He has published at leading machine learning venues (NeurIPS, ICLR, ICML, JMLR, Nature SR). He is also actively contributing to the machine learning community as an Area Chair for the leading conferences and as an Action Editor for TMLR. At Molecule.one, he leads work on developing software that understands chemistry, combining effective UI, data from in-house laboratory, and state-of-the-art deep learning models. At least when he finds the time, as he also helps build and lead the company on an operational level.
# Sponsors' Speakers
Mattia Boni Sforza is a Senior Principal Data Scientist at QuantumBlack, AI by McKinsey, in Milan. Mattia leads large teams of Data Scientists developing statistical and Machine Learning based models used in predictive, prescriptive and optimisation tasks in production systems. Mattia co-leads QB in Milan, after having spent 5 years growing QB London. His background is in Mathematics and Statistics (Imperial College, London). In his free time, he loves hiking and cycling around Italian mountains.
Dominika Kampa is an Associate Partner at QuantumBlack, AI by McKinsey, based in London. She has 6 years of experience of working with TMT players, running digital and advanced analytics transformations. She uses data and new technologies to enable the next wave of growth – often using CustomerOne, our asset for personalisation for which she also serves as a product leads. Her background is in mathematical economics (University of Warwick & Imperial College London, UK). Outside of work, she runs a handball club in East London (London GD HC) and an educational charity in Poland (UWC Poland).
Alberto Martín is an Expert Engagement Manager at QuantumBlack, AI by McKinsey, with 7+ years of professional experience in banking, fashion and renewable energies. He helps companies to leverage their data to capture its full potential, both to make them grow and to manage their risks. In addition, he is a core member of the Advanced Analytics Hub in Madrid. His background is in mathematics (Universidad de Valladolid, CSIC and Princeton University) In his free time, he loves to exercise and hang out with friends.
Jacek Szczerbiński obtained his PhD in Chemistry from ETH Zurich. He then fell in love with ML and turned a Research Engineer at Allegro. Currently, he is studying the robustness of text classifiers against mislabeled training data. His superpower is explaining ML to non-technical people.
Aleksandra Osowska-Kurczab is a Research Engineer working on recommendation systems at Allegro. Multitasking is her second name because she is also pursuing a PhD in Computer Science at Warsaw University of Technology. Her research interests include representation learning and robustness, with applications to computer vision, medical image analysis and large-scale retrieval.
Wojciech Szmyd is a data scientist at deepsense.ai with five years of experience in development of machine learning systems. He graduated in ICT from AGH University of Science and Technology, but his innate curiosity meant that he has recently begun studying philosophy at the Jagiellonian University. His professional interests are connected with natural language processing. In his free time… he has no free time, but if he had, he would travel more.
Jakub Radoszewski works as a Principal Data Scientist at Samsung R&D, Poland and as an associate professor at the University of Warsaw, Poland. He is also a Vice-Chair of the Main Committee of the Polish Olympiad in Informatics. Jakub Radoszewski received his PhD from the University of Warsaw in 2012 and habilitation in 2020. He was a Newton International Fellow at King’s College London, UK. He received the Witold Lipski Award for young researchers in computer science, best paper awards at several conferences, and has lead a few research projects on theoretical computer science. His main research area are discrete algorithms, with a focus on text algorithms. He is also interested in the interplay between algorithms and ML.
# Contributed Talks and Posters
We were very excited to invite all to submit proposals for contributed talks and posters for ML in PL ‘22 Conference!
This year we accepted 21 talks and 34 posters that will be presented during the main conference. A list of talks and posters (with slides and posters in pdf format) can be found here. Results of the Best Poster Award can be found here.
A detailed description of the Call for Contribution can be found here.
# Agenda
-
Students’ day, Friday, 4 November
# Students’ day, Friday, 4 November 2022, 09:00 - 12:00
Address:
University of Warsaw, Faculty of Mathematics, Informatics and Mechanics
Banacha 2 Street – 02-097 Warsaw
An event dedicated to students taking their first steps as researchers and speakers, consisting of a series of talks and networking sessions. More information can be found here.
Attendance at this event is free of charge!
Agenda:
09:00 - 09:05 CET: Opening remarks
09:05 - 09:25 CET: Extraction of semantic relations using selected methods and data for the Polish language (Grzegorz Kwiatkowski)
09:25 - 09:45 CET: Linear probing of transformer models for Slavic languages (Aleksandra Mysiak)
09:45 - 10:05 CET: HyperSound: Generating Implicit Neural Representations of Audio Signals with Hypernetworks (Filip Szatkowski)
10:05 - 10:25 CET: How weakening the constraints on non-negative model leads to the more practical XAI (Michał Balicki)
10:25 - 10:45 CET: Esperanto constituency parser (Tomasz Michalik)
10:45 - 11:05 CET: SurvSHAP(t): Time-dependent explanations of machine learning survival models (Mateusz Krzyziński)
11:05 - 11:25 CET: Application of Advanced Text Data Analysis in Science (Aleksandra Kowalczuk)
11:25 - 11:45 CET: Universal Image Embedding (Konrad Szafer)
11:45 - 11:50 CET: Closing remarks# Students’ day - NVIDIA Workshop, Friday, 4 November 2022, 08:00 - 14:00 (Room 4.05)
Address:
Cziitt PW
ul. Rektorska 4 00-614 Warszawa
During the workshop you will learn about the mechanics of deep learning and popular techniques, train your computer vision model and finally use transfer learning to train your own RNN model.
After the workshop you can take part in the exam, passing which you will be provided with the certificate that confirms you understood all of the topics.
Registration form: https://mlinplworkshop.paperform.co/
Note: we may close the form earlier in case we have an overwhelming number of eligible participants.For more details: https://www.nvidia.com/en-us/training/instructor-led-workshops/fundamentals-of-deep-learning/
-
Friday, 4 November
# Friday, 4 November 2022
Adress:
University of Warsaw, Auditorium Maximum
Krakowskie Przedmieście 26/28 Street - 00-927 Warszawa
-
15:00 - 15:45 CET: Registration
-
15:45 - 16:00 CET: Opening remarks
The opening remarks of the project leaders Alicja Grochocka and Dima Zhylko.
-
16:00 - 17:00 CET: Keynote Lecture: Kyunghyun Cho
Lab-in-the-loop de novo antibody design - what are we missing from machine learning?
In this talk, I will give an overview of what we do at Prescient Design by introducing the concept of lab-in-the-loop de novo antibody design with particular emphasis on its computational side. I will then deconstruct the computational side of lab-in-the-loop design into three major components; generative modeling, computational oracles and active learning. I will then go over why each component is necessary, how each component still requires significant scientific research and what Prescient Design is doing to address these challenges.
-
17:05 - 18:20 CET: Strategic Sponsor’s Lecture: QuantumBlack, AI by McKinsey
Through this series of short talks, QuantumBlack/McKinsey practitioners will share how they work across industries to push AI's limits and create solutions that transform organizations. As part of this session, you'll hear how they used Reinforcement Learning to help Emirates Team New Zealand win the 36th America's Cup. They'll also demo some of their products including Customer1, a tool that helps optimize customer journeys, and Kedro, an open-sourced Python framework for creating maintainable and modular data science code. Finally, you will hear from one of QuantumBlack's brilliant data scientists about his career journey.
-
18:35 - 19:35 CET: Panel: Popularization of ML Research
During the panel, we will discuss how we should popularize research. What should we think about when presenting AI to the public and what are some guidelines which help to reach a wider audience? We will also address the misleading presentation of scientific results.
Panelists: Piotr Migdał (Quantum Flytrap), Yannick Kilcher (DeepJudge), Letitia Parcalabescu (Heidelberg University)
-
19:40 - 20:40 CET: Keynote Lecture: Petar Veličković
Amazing things that happen with Human-AI synergy
For the past few years, I have been working on a challenging project: teaching machines to assist humans with proving difficult theorems and conjecturing new approaches to long-standing open problems. Alongside our pure mathematician collaborators from the Universities of Oxford and Sydney, we have demonstrated that analyzing and interpreting the outputs of (graph) neural networks offers a concrete way of empowering human intuition. This allowed us to derive novel top-tier mathematical results in areas as diverse as representation theory and knot theory. The significance of these results has been recognised by the journal Nature, where our work featured on the cover page. Naturally, being on a project of this scale gets one thinking: what other kinds of amazing things can one do when AI and human domain experts synergistically interact? During this talk, I will offer my personal perspective on these findings, the key details of our modelling work, and also positioning them in the "bigger picture" context of synergistic Human-AI efforts.
-
-
Saturday, 5 November
# Saturday, 5 November 2022
Address:
University of Warsaw, Faculty of Mathematics, Informatics and Mechanics
Banacha 2 Street – 02-097 Warsaw
-
09:30 - 10:00 CET: Registration
-
10:00 - 11:15 CET: Keynote Lecture: Cheng Zhang (Room 3180)
Causal Decision Making
Causal inference is essential for data-driven decision-making across domains such as business engagement, medical treatment, or policymaking. Building a framework that can answer real-world causal questions at scale is critical. However, research on deep learning, causal discovery, and inference has evolved separately. In this talk, I will present a Deep End-to-end Causal Inference (DECI) framework, a single flow-based method that takes in observational data and can perform both causal discovery and inference, including conditional average treatment effect estimation (CATE). Moreover, I will talk about how such a framework can be used with different real-world data, including time series or considering latent confounders. In the end, I will cover different application scenarios with the Microsoft causal AI suite. We hope that our work bridges the causality and deep learning communities leading to real-world impact.
-
10:00 - 11:15 CET: Keynote Lecture: Andriy Mnih (Room 4420)
Deep Generative Models Through the Lens of Inference
Latent variable modelling provides a powerful and flexible framework for generative models. We will start by introducing this framework along with the concept of inference, which is central to it. We will then cover three types of deep generative models: flow-based models, variational autoencoders, and diffusion models. We will highlight the interdependence between the model structure and the inference process, and explain the trade-offs each model type involves.
-
11:30 - 12:20 CET: Sponsor’s Talk: deepsense.ai (Room 3180)
TrelBERT – a model that understands the language of social media
Wojciech Szmyd, Data Scientist (deepsense.ai)Abstract: With the growing importance and popularity of social media, the application of natural language processing techniques to this domain is becoming an increasingly trendy topic both among NLP researchers and practitioners. In this talk I will explain what is so interesting in the language of social media and why this topic requires special attention. deepsense.ai actively follows the latest international developments in the field of NLP and as part of an R&D project has introduced TrelBERT - a language model for Polish social media which has achieved outstanding results in detecting cyberbullying.
Speaker's bio: Wojtek Szmyd is a data scientist at deepsense.ai with five years of experience in development of machine learning systems. He graduated in ICT from AGH University of Science and Technology, but his innate curiosity meant that he has recently begun studying philosophy at the Jagiellonian University. His professional interests are connected with natural language processing. In his free time… he has no free time, but if he had, he would travel more.
-
11:30 - 12:20 CET: Contributed Talks Session 1: Learning with Positive and Unlabeled Data (Room 4420)
One class classification approach to variational learning from Positive Unlabeled Data
Jan Mielniczuk (Institute of Computer science, PAS and Warsaw University of Technology)We present Empirical Risk Minimization approach in conjunction with variational inference method to learn classifiers for biased Positive Unlabeled data. Labeled data is biased meaning that labeling depends on covariates and thus the labeled data is not a random sample from a positive class. This extends method VAE-PU introduced by Na et al. (2020, ACM IKM Conference Proceedings) by proposing another estimator of theoretical risk of a classifier to be minimized, which has important advantages over the previous proposal. This is based on one class classification approach which turns out to be an effective method of detecting positive samples among unlabeled ones. Experiments performed on real data sets show that the proposed VAE-PU+OCC algorithm works very promisingly in comparison to its competitors such as the original VAE-PU and SAR-EM method in terms of accuracy and F1 score.
How to learn classifier chains using positive-unlabelled multi-label data?
Paweł Teisseyre (Institute of Computer Science, Polish Academy of Sciences and Faculty of Mathematics and Information Sciences, Warsaw University of Technology)Multi-label learning deals with data examples which are associated with multiple class labels simultaneously. The problem has attracted significant attention in recent years and dozens of algorithms have been proposed. However in traditional multi-label setting it is assumed that all relevant labels are assigned to the given instance. This assumption is not met in many real-world situations. In positive unlabelled multi-label setting, only some of relevant labels are assigned. The appearance of a label means that the instance is really associated with this label, while the absence of the label does not imply that this label is not proper for the instance. For example, when predicting multiple diseases in one patient, some diseases can be undiagnosed however it does not mean that the patient does not have these diseases. Among many existing multi-label methods, classifier chains gained the great popularity mainly due to their simplicity and high predictive power. However, it turns out that adaptation of classifier chains to positive unlabelled framework is not straightforward, due to the fact that the true target variables are observed only partially and therefore they cannot be used directly to train the models in the chain. The partial observability concerns not only the current target variable in the chain but also the feature space, which additionally increases the difficulty of the problem. We propose two modifications of classifier chains. In the first method we scale the output probabilities of the consecutive classifiers in the chain. In the second method we minimize weighted empirical risk, with weights depending on prior probabilities of the target variables. The predictive performance of the proposed methods is studied on real multi-label datasets for different positive unlabelled settings.
-
11:30 - 12:20 CET: Contributed Talks Session 2: Computer Vision (Room 5440)
NeRF – Generating 3d world from a bunch of images
Łukasz Pierścieniewski (NVIDIA)Neural radiance fields (NeRFs) are an emerging area in computer vision research that focuses on generating novel views of complex 3D scenes based on a partial set of 2D images. Instead of optimizing model parameters to perform decision-based tasks on unseen data, the model learns the representation of the given sample within its parameters. This allows it to freely generate object renders from different angles and positions based on this learned representation. The results are striking, but the depth maps produced as a side effect are even more useful. NeRF provides practitioners with the ability to model virtual worlds through scenes from the physical world, or insert virtual objects in correct places in augmented reality environments. In this talk we are going to dive into this technology, its current state-of-the-art approaches, and open challenges for the future
How to win MAI'2022 Depth Estimation challenge: manual backbone optimization combined with smart model pruning
Łukasz Treszczotko (TCL Research Europe)Mobile AI challenge is an international competition organized in connection with CVPR'2022 and ECCV'2022 conferences, where teams from best universities and companies are supposed to optimize AI models for practical deployments. Here, both model quality and execution time should be jointly optimized for target low-power hardware platforms. Amongst 5 tasks, we took part in the Depth Estimation challenge evaluated on the Raspberry Pi 4 device and we managed to win the competition. In this talk, we show the techniques that led us to this success. We demonstrate our in-house developed neural model compression framework that contains 1) a pruning algorithm that learns channel importance directly from feature maps; 2) an algorithm that groups the interdependent blocks of channels and 3) device-aware pruning modes that can be adjusted to specific hardware platforms. Using this frameworks we have been able to successfully compress a wide range of backbone models, e.g., EfficientNet family, MobileNetV3 family, ViT-based backbones for classification as well as networks for semantic segmentation, denoising, detection, depth estimation, to name a few. This fully automated pruning technique was combined with manual backbone adjustments and some other tricks described during the talk. As a result, we got the highest quality rating of all competitors and the second-fastest model execution time on Raspberry Pi device (46 ms), beating the second-best candidate by almost 30% in the final score. We will also briefly discuss real-world applications of model pruning and depth estimation models on TCL smartphones.
-
12:20 - 13:30 CET: Lunch Break
-
13:30 - 14:20 CET: Sponsor’s Talk: Amazon (Room 3180)
Challenges and evolution of Alexa Text-To-Speech
Adam Furmanek, Senior Software Engineer (Amazon)Abstract: It was quite a hike to deliver a first world-class voice assistant, but nowadays it is common to control computers with your voice. What about the future? What else is there in the world of Text-To-Speech? In this talk we are going to see what challenges Alexa TTS is facing now. We’ll go through the history of TTS, technical solutions, and then focus on what future brings. We’ll focus on both technical and business problems we’re solving now, and how these things will affect our lives.
Speaker's bio: Adam Furmanek is a Senior Software Engineer at Alexa. He works on the backend side of Alexa Text-To-Speech. He specializes in machine learning and large scale distributed systems. In his free time, he watches The Office and plays piano.
-
13:30 - 14:20 CET: Contributed Talks Session 3: Probabilistic & Auto Machine Learning (Room 4420)
Interactive sequential analysis of a model improves the performance of human decision-making
Hubert Baniecki (University of Warsaw)Evaluation of explainable machine learning, especially with human subjects, became mandatory for the trustworthy adoption of predictive models in various applications. This contribution focuses on reporting the results from a user study considering evaluating model explanations in a real-world medical use case; as described in Section 5 of the paper: Baniecki, D. Parzych, P. Biecek. The Grammar of Interactive Explanatory Model Analysis, 2022 (https://arxiv.org/abs/2005.00497v4). The contribution's title is mainly supported by Tables 4 & 5. Moreover, we find both: the user study design and the IEMA grammar's theoretical background worth discussing.
Lightweight Conditional Model Extrapolation for Streaming Data under Class Prior Shift (LIMES)
Paulina Tomaszewska (Warsaw University of Technology)Many Machine Learning models don’t work well under large class imbalance. The problem gets even more difficult when the class distribution changes over time. It may happen in non-stationary data streams. We address this issue in the proposed method called LIMES which stands for Lightweight Model Extrapolation for Streaming data under Class-Prior Shift. The model works in a continuous manner. It is lightweight as it adds no trainable parameters and almost no memory or computational overhead compared to training a single model. The solution is inspired by the MAML network where one model that can be easily adjusted to different scenarios. In the case of MAML, the adaptation is done using few gradient updates, in the case of LIMES, we apply analytical formula derived from the Bayesian rule. The core of the method is bias correction term that allows to shift from the reference class distribution to the target one. At this point, the extrapolation step is also needed in the case when the exact target class distribution is not a priori known. We evaluated our method on Twitter data where the goal was to find out the country where the tweet was issued. The results that we got show that LIMES outperforms baselines especially in the most difficult scenarios.
-
13:30 - 14:20 CET: Contributed Talks Session 4: Deep Learning (Room 5440)
Introduction and analyzing of Diffusion-based Deep Generative Models
Kamil Deja (Warsaw University of Technology)Diffusion-based Deep Generative Models (DDGMs) offer state-of-the-art performance in generative modeling. Their main strength comes from their unique setup in which a model (the backward diffusion process) is trained to reverse the forward diffusion process, which gradually adds noise to the input signal. Although DDGMs are well studied, it is still unclear how the small amount of noise is transformed during the backward diffusion process. In this talk we will introduce the general idea behind Diffusion-based generative models while focusing on denoising and generative capabilities of those models. We observe a fluid transition point that changes the functionality of the backward diffusion process from generating a (corrupted) image from noise to denoising the corrupted image to the final sample. Based on this observation, we postulate to divide a DDGM into two parts: a denoiser and a generator. The denoiser could be parameterized by a denoising auto-encoder, while the generator is a diffusion-based model with its own set of parameters. We experimentally validate our proposition, showing its pros and cons.
Session-aware Graph Neural Network-based Recommendations for Custom Advertisement Segment Generation Focused on Game players (remote)
Tomasz Palczewski (Samsung Research America)Recommendation engines are commonly used across a broad range of verticals. One can find these solutions in e-commerce, advertisement, marketing, news, on TVs, and within streaming service content. They are extremely important to keep a high-level user engagement when users face an overwhelming number of items that can be potentially presented to them. In general, recommendation systems can be divided into two groups - 1) systems built on general interaction information and 2) systems built on sequential interaction data. As the field has progressed, the research has revolved around expanding both groups to include different types of auxiliary information. The sequence-based recommendation systems can be categorized as session-based solutions that rely on the user's actions in the current sessions. The session-aware recommendation systems also leverage user information in the historical sessions. In this paper, we propose a novel Session-awaRe grAph Neural Network-based Recommendation model (called SRA-NN-Rec for brevity) and test it using publicly available datasets as well as present its usefulness in a real-word case for custom advertisement segment generation focused on game players. The key components of SRA-NN-Rec are: 1) a graph neural network that consumes user and session information, 2) a self-attention network to enhance item embeddings based on session data, and 3) an attention network. The experiment results show that the SRA-NN-Rec outperforms the existing baseline models and is an effective model for session-aware recommendations.
-
14:35 - 15:50 CET: Panel: Women in ML in PL (Room 3180)
This event will see a discussion on participants’ research topics, their career paths and their views on current ML trends.
Panelists: Farah Shamout (NYU Abu Dhabi) , Inez Okulska (NASK National Research Institute), Aga Dobrowolska (Relation Therapeutics), Daria Szmurlo (QuantumBlack, AI by McKinsey)
-
14:35 - 15:50 CET: Contributed Talks Session 5: NCBR IDEAS (Room 4420)
Improved Feature Importance Computation for Tree Models Based on the BanzhafValue
Piotr Sankowski (IDEAS NCBR, MIM Solutions and University of Warsaw)The Shapley value -- a fundamental game-theoretic solution concept -- has recently become one of the main tools used to explain predictions of tree ensemble models. Another well-known game-theoretic solution concept is the Banzhaf value. Although the Banzhaf value is closely related to the Shapley value, its properties w.r.t. feature attribution have not been understood equally well. This paper shows that, for tree ensemble models, the Banzhaf value offers some crucial advantages over the Shapley value while providing similar feature attributions.
In particular, we first give an optimal O(TL+n) time algorithm for computing the Banzhaf value-based attribution of a tree ensemble model's output. Here, T is the number of trees, L is the maximum number of leaves in a tree, and n is the number of features. In comparison, the state-of-the-art Shapley value-based algorithm runs in O(TLD^2+n) time, where D denotes the maximum depth of a tree in the ensemble.
Next, we experimentally compare the Banzhaf and Shapley values for tree ensemble models. Both methods deliver essentially the same average importance scores for the studied datasets using two different tree ensemble models (the sklearn implementation of Decision Trees or xgboost implementation of Gradient Boosting Decision Trees). However, our results indicate that, on top of being computable faster, the Banzhaf is more numerically robust than the Shapley value.
Interpretable Deep Learning with Prototypes
Bartosz Zieliński (IDEAS, Jagiellonian University)The broad application of deep learning in fields like medical diagnosis and autonomous driving enforces models to explain the rationale behind their decisions. That is why explainers and self-explainable models are developed to justify neural network predictions. Some of them are inspired by mechanisms used by humans to explain their decisions, like matching image parts with memorized prototypical features that an object can possess. Recently, a self-explainable model called Prototypical Part Network (ProtoPNet) was introduced, employing feature matching learning theory. It focuses on crucial image parts and compares them with reference patterns (prototypical parts) assigned to classes.
In this presentation, we will present our papers concerning this topic published on the main track of three recent conferences (SIGKDD 2021, ECML PKDD 2022, and ECCV 2022). They discuss the topic of prototype sharing between classes and their adaptation to the weakly-supervised setting.
Fine-Grained Conditional Computation in Transformers
Sebastian Jaszczur (IDEAS NCBR, University of Warsaw)Large Transformer models yield impressive results on many tasks, but are expensive to train, or even fine-tune, and so slow at decoding that the use and study of the largest models becomes out of reach for many researchers and end-users. Conditional computation, or sparsity, may help alleviate those problems.
In my work "Sparse is Enough in Scaling Transformers", done at Google Research and published at NeurIPS 2021, we showed that sparse layers leveraging fine-grained conditional computation can enable Transformers to scale efficiently and perform unbatched decoding much faster than standard Transformer. Importantly, in contrast to standard Mixture-of-Expert methods, this fine-grained sparsity achieves the speed-up without decreasing the model quality, and with the same number of model parameters.
My current work on this topic, done at IDEAS NCBR, focuses on adjusting those conditional computation methods to the training environment, with the goal of speeding up the training process as well as the inference. This can be achieved by a careful redesign of fine-grained conditional computation while using only dense tensor operations, which are efficient on modern accelerators. While this is still an ongoing work, the preliminary results show the promise of improving the training speed of Transformers on existing hardware, without degrading the quality of the model's predictions.
-
14:35 - 15:50 CET: Contributed Talks Session 6: Reinforcement Learning (Room 5440)
Experimental Evaluation of Fictitious Self-play Algorithm in Unity Dodgeball Environment
Jacek Cyranka (University of Warsaw)We introduce the generalized fictitious co-play algorithm (GFCP) for team video games. Our algorithm constitutes two phases and can be applied on top of any multi-agent RL training procedure in a setting of a simultaneous team game. First, a pool of checkpoints is trained using a vanilla self-play algorithm. Second, further training is carried out via fictitious co-play, i.e., setting one of the agents to inference mode and sampling previously frozen checkpoints. We evaluate our algorithm in the Unity Dodgeball elimination 4vs4 environment. The experiments show the favorable properties of GFCP agents in terms of win rate when matched with weaker checkpoints and previously unseen checkpoints that were not used for the training. On top of that, we evaluate the capability of the GFCP agents to match in a team with human players within a hand-crafted computer game built in the Unity environment.
Fast and Precise: Adjusting Planning Horizon with Adaptive Subgoal Search
Michał Tyrolski (University of Warsaw)Complex reasoning problems contain states that vary in the computational cost required to determine a good action plan. Taking advantage of this property, we propose Adaptive Subgoal Search (AdaSubS), a search method that adaptively adjusts the planning horizon. To this end, AdaSubS generates diverse sets of subgoals at different distances. A verification mechanism is employed to filter out unreachable subgoals swiftly and thus allowing to focus on feasible further subgoals. In this way, AdaSubS benefits from the efficiency of planning with longer subgoals and the fine control with the shorter ones. We show that AdaSubS significantly surpasses hierarchical planning algorithms on three complex reasoning tasks: Sokoban, the Rubik's Cube, and inequality proving benchmark INT, setting new state-of-the-art on INT.
Reinforcement learning in Datacenters
Alessandro Seganti (Equinix)Did you know that datacenters are a highly controlled environment? That inside a datacenter we have thousands of PID controllers that are automating every aspect of the functioning? This together with Equinix expertise, allows our datacenters to operate without flaws (99.9999% uptime record).
In our presentation we share our experience in using reinforcement learning in a datacenter. Hopefully by the end of the presentation you will learn what are the challenges and advantages of using reinforcement learning in a real world scenario.
During this presentation we will show how data centers are controlled. We will also introduce you to the kind of data we are using and how we are processing it. Then we will explain how we used reinforcement learning to improve the energy efficiency of a simulated datacenter. Finally we will show how our experiments and models have been used in real use cases inside the company.
-
16:05 - 17:20 CET: Contributed Talks Session 7: Natural Language Processing (Room 3180)
Automated Harmful Content Detection Using Grammar-Focused Representations of Text Data
Daria Stetsenko (NASK PIB)Harmful content detection is one of the most important topics in natural language processing. Every couple of years, surveys and thesis are published that summarize the state-of-the-art methods to approach the problem. In 2019 even one of the shared tasks in SemEval Competition focused solely on offensive language detection. Both classical and neural models have been proposed and compared in the literature, with different levels of analysis: the lexical level (including sentiment analysis), the meta-information (context), and, if applicable, the multimodal perspective. However, almost all the mentioned models relied on semantic word or sentence embeddings, putting aside the potential of grammar. And there is a lot of meaningful information to be extracted from the grammar layer.
This research project aims to find models to extract and process the grammar indicators of harmful content in written texts, and investigate possible ways of representing them, most probably combined with the lexical ones. The research includes work on sentence and document embeddings that preserve linguistic information interpretable for both machine-learning algorithms and humans. We strive to set the clear-cut linguistic boundaries of syntactic constructions and semantic features for the categorization of harmful content, defined as a general category including hate speech, violence description, and pornography. The goal would be to determine a linguistic representation of each of them.
Building more helpful and safe dialogue agents via targeted human judgements
Maja Trębacz (DeepMind)We present Sparrow, an information-seeking dialogue agent trained to be more helpful, correct, and harmless compared to prompted language model baselines. We use reinforcement learning from human feedback to train our models with two new additions to help human raters judge agent behavior. First, to make our agent more helpful and harmless, we break down the requirements for good dialogue into natural language rules the agent should follow, and ask raters about each rule separately. We demonstrate that this breakdown enables us to collect more targeted human judgements of agent behavior and allows for more efficient rule-conditional reward models. Second, our agent provides evidence from sources supporting factual claims when collecting preference judgements over model statements. For factual questions, evidence provided by Sparrow supports the sampled response 78% of the time. Sparrow is preferred more often than baselines while being more resilient to adversarial probing by humans, violating our rules only 8% of the time when probed. Finally, we conduct extensive analyses showing that though our model learns to follow our rules it can exhibit distributional biases.
Blogpost: https://dpmd.ai/sparrow, tech report: https://dpmd.ai/sparrow-paper
Uncertainty estimation in BERT-based Named Entity Recognition
Łukasz Rączkowski (Allegro Machine Learning Research)Named Entity Recognition (NER) is one of the standard tasks in natural language processing and models optimized for it are routinely deployed for users. With the advent of deep learning in general, and Transformer-based architectures in particular, the SOTA for NER moved towards large parameter spaces. However, such models are known to be poorly calibrated black boxes, which do not provide any uncertainty estimates. These estimates are crucial for misclassification detection and out-of-distribution detection in deployed machine learning models. Despite the common application of NER, the issue of uncertainty estimation in that task is not well studied as of yet. This talk will cover a novel exploration of NER uncertainty estimation in the context of e-commerce product descriptions.
We pre-trained a BERT-based language model on our internal corpus of around 35 million product descriptions. We then used that pre-trained backbone to fine-tune NER classifiers in several product categories and utilized the variational dropout technique to measure the predictive entropy for each observation, which served as a general-purpose uncertainty metric.
With variational dropout, we were able to properly calibrate our NER models and the entropy uncertainty measure proved to be a good indicator of both misclassified and out-of-distribution observations. Moreover, thanks to the effective model averaging provided by variational dropout, we improved our internal NER evaluation metrics. The solution discussed in this talk is a relatively simple method of uncertainty estimation and can be effectively utilized in production-grade NER models.
-
16:05 - 17:20 CET: Remote Keynote Lecture: Marc Deisenroth (Room 4420, Remote)
Data-Efficient Machine Learning in Robotics
Data efficiency, i.e., learning from small datasets, is of practical importance in many real-world applications and decision-making systems. Data efficiency can be achieved in multiple ways, such as probabilistic modeling, where models and predictions are equipped with meaningful uncertainty estimates, Bayesian optimization, transfer learning, or the incorporation of valuable prior knowledge. In this talk, I will focus on how robot learning can benefit from data-efficient learning algorithms. In particular, I will motivate the use of Gaussian process models for learning good policies in three different settings: model-based reinforcement learning, transfer learning, and Bayesian optimization.
-
16:05 - 17:20 CET: Remote Keynote Lecture: Aleksander Mądry (Room 5440, Remote)
Datamodels: Predicting Predictions with Training Data
Machine learning models tend to rely on an abundance of training data. Yet, understanding the underlying structure of this data - and models' exact dependence on it - remains a challenge.
In this talk, we will present a new framework - called datamodeling - for directly modeling predictions as functions of training data. This datamodeling framework, given a dataset and a learning algorithm, pinpoints - at varying levels of granularity - the relationships between train and test point pairs through the lens of the corresponding model class. Even in its most basic version, datamodels enable many applications, including discovering subpopulations, quantifying model brittleness via counterfactuals, and identifying train-test leakage.
-
17:20 - 19:00 CET: Poster Session (Room 2180)
During this session, the authors will present their posters. We accepted 34 posters this year. A complete list of accepted posters can be found here.
-
17:25 - 17:50 CET: Lightning Talk: Samsung (Room 3180)
CompactAlign: A Tool for Large-Scale Near-Duplicate Text Alignment
Jakub Radoszewski (Samsung)
Detecting near-duplicate passages between a collection of source documents and a query document constitutes one of the crucial steps of plagiarism detection. This problem is computation-intensive, especially for long documents. Due to the high computation cost, many solutions primarily rely on heuristic rules, such as the "seeding-extension-filtering" pipeline, and involve many hard-to-tune hyper-parameters. We will describe an efficient tool for detecting near-duplicate passages called TxtAlign that was developed recently by Deng et al. (SIGMOD’21, SIGMOD’22) and a new tool, CompactAlign, that can be seen as a compact version of TxtAlign. Both tools use bottom-k sketching for approximate Jaccard index to assess the similarity measure of passages. CompactAlign outperforms TxtAlign in terms of both space usage and query time by orders of magnitude. The presentation will be partially based on joint work with Roberto Grossi, Costas S. Iliopoulos, and Zara Lim.
-
20:00 - ? CET: Conference Party
After a long day of talks, panels, and sessions, you can relax and network at the conference party. It will be hosted at River Club (https://goo.gl/maps/wNgp3qZxkCdJigor9) with pizza, finger food, drinks, and music included. At the entrance, every participant will receive a wristband.
-
-
Sunday, 6 November
# Sunday, 6 November 2022
Address:
University of Warsaw, Faculty of Mathematics, Informatics and Mechanics
Banacha 2 Street – 02-097 Warsaw
-
9:30 - 10:00 CET: Registration
-
10:00 - 11:15 CET: Keynote Lecture: Matthias Bethge (Room 3180)
Brain-like visual representations, decision making, and learning
Representations, decision making, and learning are closely interlinked components of intelligent systems that can be studied both in brains and machines. Since the advent of large datasets and extensive use of machine learning in computer vision, the range of tasks that machines can solve (often better than humans) has been rapidly growing. However, both the type of "knowledge" and the way how it is acquired, still seems fundamentally different between brains and machines, and the relation between neuroscience and machine learning is a tricky one. In this talk, I will present my own approach to research at the intersection of the two fields and argue that the emerging field of lifelong machine learning will be key to bringing the two fields closer together in the future.
-
10:00 - 11:15 CET: Keynote Lecture: Piotr Miłoś (Room 4420)
Sequential decision-making: its promises and challenges
Intelligence can be defined as the ability to set and achieve goals. To do this, an agent needs to execute a prolonged sequence of actions. Making an intelligent decision on which action to choose requires anticipating its future consequences and an accurate perception of the current state. Both tasks are cognitively demanding, even more so if the actions are to be swiftly rendered under computational or time constraints. The key to acquiring such skills is to learn them, leveraging past experiences and often deep learning techniques. Sequential decision-making techniques can be used to control complex systems. In my talk, I will illustrate what can be achieved with a few examples. Further, I will outline a few promising future research directions and related challenges.
-
11:30 - 12:20 CET: Sponsor’s Talk: Allegro (Room 3180)
Retrieval At Scale
We will show how we operate retrieval in different areas of the company by applying a diverse set of techniques that allow our models to run big and fast. In the presentation, we will show how one could tackle the problem of retrieval in the e-commerce domain using state-of-the-art deep learning models.
-
11:30 - 12:20 CET: Contributed Talks Session 8: Science-related ML (Room 4420)
From data acquisition to ML model for predicting outcomes of chemical reactions
Michał Sadowski (Molecule.one)Outcomes of many chemical reactions are hard to predict for humans and computers alike. Despite significant progress in computational chemistry, we are still far from replacing the experiment with simulations. One of the promising approaches to predicting reaction outcomes is based on deep neural networks. It is however fundamentally limited by available data, which is especially problem in chemistry in which datasets of reactions are not diverse and consist almost only of positive reactions. In order to demonstrate commercially-viable data-driven learning about chemical reactions, we designed a methodology based on the data acquired in our own high-throughput lab. We tested out and used different active learning techniques for choosing which reactions should be performed. We also examined applicability (is input sufficiently similar to the reactions it saw during training) and uncertainty (how certain the model is when determining prediction) metrics for our deep learning models. In order to ensure usability and reliability of our models, we kept chemist-in-the-loop in all phases of the project. In this talk we will discuss challenges in building real world machine learning system from data acquisition, designing ML models to its final evaluation.
Sequential counterfactual prediction to support individualized decisions on treatment initiation
Paweł Morzywołek (Department of Applied Mathematics, Computer Science and Statistics, Ghent University)Digitalization of patient records and increasing computational power have led to a paradigm shift in the field of medical decision-making from one-size-fits-all interventions to data-driven intervention strategies optimised for particular sub-populations or individuals. In this research, we make use of statistical techniques on counterfactual prediction and heterogeneous treatment effects (CATE) estimation to support patient-centered treatment decisions on the initiation of renal replacement therapy (RRT).
In particular, we applied sequential counterfactual prediction strategies to help physicians in making patient-centered decisions on treatment initiation. For this, we made use of linked databases from the Intensive Care Unit (ICU) and dialysis center of the Ghent University Hospital, containing longitudinal, highly granular records from all adult patients admitted to the ICU from 2013 to 2017. Based on these data, we inferred whether or not to initiate RRT for individual patients suffering acute kidney injury (AKI) on each day of their ICU stay, based on their measurements until that day.
Because RRT is not a feasible treatment option for many patients, standard counterfactual prediction strategies are fallible. In our analysis we therefore study the use of retargeted causal learning techniques, which reweight individuals to generate a population of patients for whom all treatment options are plausible, so that counterfactual prediction becomes feasible. The proposed approach leverages recent developments on orthogonal statistical learning literature, resulting in a class of Neyman-orthogonal weighted loss functions for counterfactual prediction. Our approach is guaranteed to deliver predictions in the support of the counterfactual outcome mean, and delivers oracle behavior due to orthogonality of the loss function (where orthogonality is relative to the infinite-dimensional propensity score and conditional outcome mean).
Our study involves a performance evaluation of state-of-the-art methods from the literature on heterogeneous treatment effects estimation, e.g. DR-Learner and R-Learner, which can be viewed as special cases resulting from the proposed retargeting framework for counterfactual prediction and CATE estimation.
-
11:30 - 12:20 CET: Contributed Talks Session 9: Probabilistic Neural Networks and Consolidated Learning (Room 5440)
Towards self-certified learning: Probabilistic neural networks trained by PAC-Bayes with Backprop
Omar Rivasplata (University College London)This talk is based on the content of my paper "Tighter Risk Certificates for Neural Networks" (JMLR, 2021) which reports the results of empirical studies regarding training probabilistic (aka stochastic) neural networks using training objectives derived from PAC-Bayes bounds. In this context, the output of training is a probability distribution over network weights, rather than a fixed setting of the weights. We present two training objectives, used for the first time in connection with training neural networks, which were derived from tight PAC-Bayes bounds. We also re-implement a previously used training objective based on a classical PAC-Bayes bound, to compare the properties of the predictors learned using the different training objectives. We compute risk certificates for the learnt predictors, which are valid on unseen data from the same distribution that generated the training data. The risk certificates are computed using part of the data used to learn the predictors. We further experiment with different types of priors on the weights (both data-free and data-dependent priors) and neural network architectures. Our experiments on MNIST and CIFAR-10 show that our training methods produce stochastic neural network classifiers with competitive test set errors and non-vacuous risk bounds with much tighter values than previous results in the literature. Thus, our results show promise not only to guide the learning algorithm through bounding the risk but also for model selection. These observations suggest that the methods studied in our work might be good candidates for self-certified learning, in the sense of using the whole data set for learning a predictor and certifying its performance with a risk certificate that is valid on unseen data (from the same distribution as the training data) and reasonably tight, so that the certificate value is informative of the value of the true error on unseen data.
Consolidated learning – new approach to domain-specific strategy of hyperparameter optimization
Katarzyna Woźnica (Mi2, Faculty of Mathematics and Information Science, Warsaw University of Technology)For many machine learning models, a choice of hyperparameters is a crucial step towards achieving high performance. Prevalent meta-learning approaches focus on obtaining good hyperparameters configurations with a limited computational budget for a completely new task based on the results obtained from the prior tasks. In this presentation, I will present a new formulation of the tuning problem, called consolidated learning, more suited to these practical challenges faced by ML developers creating models on similar datasets. In domain-specific ML applications, ones do not solve a single prediction problem, but a whole collection of them, and their data sets are composed of similar variables. In such settings, we are interested in the total optimization time rather than tuning for a single task. Consolidated learning assumes leveraging these relations and supporting meta-learning approaches. Providing the benchmark metaMIMIC, we show that consolidated learning enables an effective hyperparameter transfer even in a model-free optimization strategy. In the talk, we will show that the potential of consolidated learning is considerably greater due to its compatibility with many machine learning application scenarios. We investigate the extension of the application of consolidated learning through integrating diverse data sets using the ontology-based similarity of data sets.
-
12:20 - 13:30 CET: Lunch Break
-
13:30 - 14:45 CET: Panel: Industry and Academia (Room 3180)
During the panel, we will investigate the wide range of implications of artificial intelligence (AI), and delve deep into both positive and negative impacts on communities and science areas, and industry.
Panelists: Przemysław Biecek (Warsaw University of Technology and University of Warsaw), Robert Bogucki(deepsense.ai), Pablo Ribalta(Nvidia), Piotr Sankowski (IDEAS NCBR), Stanisław Jastrzębski (molecule.one)
-
13:30 - 14:45 CET: Keynote Lecture: Farah Shamout (Room 4420)
Towards Improved Health via Computational Precision Medicine: Learning from Diverse and Heterogeneous Data
In the era of big data and artificial intelligence, computational precision medicine can create opportunities that directly contribute to saving patient lives, improving efficiencies of care providers, and decreasing the high burden of disease and socioeconomic costs. In this talk, I will provide a high-level overview of the research conducted at the Clinical Artificial Intelligence Lab at NYU Abu Dhabi. I will then focus on one recent flagship project related to breast cancer detection in ultrasound imaging using deep learning and 5 million ultrasound images. Finally, I will describe the themes of ongoing work, in order to advance patient diagnosis and prognosis and promote multidisciplinary contributions in computing and health sciences in the region.
-
14:45 - 15:15 CET: Closing remarks (Room 3180)
The closing remarks of the project leaders Alicja Grochocka and Dima Zhylko.
-
# Previous Editions
# Scientific Board
Ewa Szczurek is an assistant professor at the Faculty of Mathematics, Informatics and Mechanics at the University of Warsaw. She holds two Master degrees, one from the Uppsala University, Sweden and one from the University of Warsaw, Poland. She finished PhD studies at the Max Planck Institute for Molecular Genetics in Berlin, Germany and conducted postdoctoral research at ETH Zurich, Switzerland. She now leads a research group focusing on machine learning and molecular biology, with most applications in computational oncology. Her group works mainly with probabilistic graphical models and deep learning, with a recent focus on variational autoencoders.
Henryk Michalewski obtained his Ph.D. in Mathematics and Habilitation in Computer Science from the University of Warsaw. Henryk spent a semester in the Fields Institute, was a postdoc at the Ben Gurion University in Beer-Sheva and a visiting professor in the École normale supérieure de Lyon. He was working on topology, determinacy of games, logic and automata. Then he turned his interests to more practical games and wrote two papers on Morpion Solitaire. Presenting these papers at the IJCAI conference in 2015 he met researchers from DeepMind and discovered the budding field of deep reinforcement learning. This resulted in a series of papers including Learning from memory of Atari 2600, Hierarchical Reinforcement Learning with Parameters, Distributed Deep Reinforcement Learning: Learn how to play Atari games in 21 minutes and Reinforcement Learning of Theorem Proving.
Jacek Tabor in his scientific work deals with broadly understood machine learning, in particular with deep generative models. He is also a member of the GMUM group (gmum.net) aimed at popularization and development of machine learning methods in Cracow.
Jan Chorowski is an Associate Professor at Faculty of Mathematics and Computer Science at University of Wrocław. He received his M.Sc. degree in electrical engineering from Wrocław University of Technology and Ph.D. from University of Louisville. He has visited several research teams, including Google Brain, Microsoft Research and Yoshua Bengio’s lab. His research interests are applications of neural networks to problems which are intuitive and easy for humans and difficult for machines, such as speech and natural language processing.
Prior to joining Yahoo! Research Krzysztof Dembczyński was an Assistant Professor at Poznan University of Technology (PUT), Poland. He has received his PhD degree in 2009 and Habilitation degree in 2018, both from PUT. During his PhD studies he was mainly working on preference learning and boosting-based decision rule algorithms. During his postdoc at Marburg University, Germany, he has started working on multi-target prediction problems with the main focus on multi-label classification. Currently, his main scientific activity concerns extreme classification, i.e., classification problems with an extremely large number of labels. His articles has been published at the premier conferences (ICML, NeurIPS, ECML) and in the leading journals (JMLR, MLJ, DAMI) in the field of machine learning. As a co-author he won the best paper award at ECAI 2012 and at ACML 2015. He serves as an Area Chair for ICML, NeurIPS, and ICLR, and as an Action Editor for MLJ.
Krzysztof Geras is an assistant professor at NYU School of Medicine and an affiliated faculty at NYU Center for Data Science. His main interests are in unsupervised learning with neural networks, model compression, transfer learning, evaluation of machine learning models and applications of these techniques to medical imaging. He previously completed a postdoc at NYU with Kyunghyun Cho, a PhD at the University of Edinburgh with Charles Sutton and an MSc as a visiting student at the University of Edinburgh with Amos Storkey. His BSc is from the University of Warsaw. He also completed industrial internships in Microsoft Research (Redmond and Bellevue), Amazon (Berlin) and J.P. Morgan (London).
Marek Cygan is currently an associate professor at the University of Warsaw, leading a newly created Robot learning group, focused on robotic manipulation and computer vision. Additionally, CTO and co-founder of Nomagic, a startup delivering smart pick-and-place robots for intralogistics applications. Earlier doing research in various branches of algorithms, having an ERC Starting grant on the subject.
Piotr Miłoś is an Associate Professor at the Faculty of Mathematics, Mechanics and Computer Science of the University of Warsaw. He received his Ph.D. in probability theory. From 2016 he has developed interest in machine learning. Since then he collaborated with deepsense.ai on various research projects. His focus in on problems in reinforcement learning.
Przemysław Biecek obtained his Ph.D. in Mathematical Statistics and MSc in Software Engineering at Wroclaw University of Science and Technology. He is currently working as an Associate Professor at the Faculty of Mathematics and Information Science, Warsaw University of Technology, and an Assistant Professor at the Faculty of Mathematics, Informatics and Mechanics, University of Warsaw.
Razvan Pascanu is a Research Scientist at Google DeepMind, London. He obtained a Ph.D. from the University of Montreal under the supervision of Yoshua Bengio. While in Montreal he was a core developer of Theano. Razvan is also one of the organizers of the Eastern European Summer School. He has a wide range of interests around deep learning including optimization, RNNs, meta-learning and graph neural networks.
Tomasz Trzciński is an Associate Professor at Warsaw University of Technology since 2015, where he leads a Computer Vision Lab. He was a Visiting Scholar at Stanford University in 2017 and at Nanyang Technological University in 2019. Previously, he worked at Google in 2013, Qualcomm in 2012 and Telefónica in 2010. He is an Associate Editor of IEEE Access and MDPI Electronics and frequently serves as a reviewer in major computer science conferences (CVPR, ICCV, ECCV, NeurIPS, ICML) and journals (TPAMI, IJCV, CVIU). He is a Senior Member of IEEE and an expert of National Science Centre and Foundation for Polish Science. He is a Chief Scientist at Tooploox and a co-founder of Comixify, a technology startup focused on using machine learning algorithms for video editing.
Viorica Patraucean is a research scientist in DeepMind. She obtained her PhD from University of Toulouse on probabilistic models for low-level image processing. She then carried out postdoctoral work at Ecole Polytechnique Paris and University of Cambridge, on processing of images, videos, and point-clouds. Her main research interests revolve around efficient vision systems, with a focus on deep video models. She is one of the main organisers of EEML summer school and has served as program committee member for top Computer Vision and Machine Learning conferences.
# ML in PL Association
We are a group of young people who are determined to bring the best of Machine Learning to Central and Eastern Europe by creating a high-quality event for every ML enthusiast. Although we come from many different academic backgrounds, we are united by the common goal of spreading the knowledge about the discipline.
Learn more about ML in PL Association# Organizers
# Volunteers
Aleksandra Możwiłło
Andrzej Pióro
Artur Gajowniczek
Dominik Koterwa
Filip Szympliński
Igor Kołakowski
Igor Urbanik
Jan Fidor
Julia Kahan
Kamila Kopacz
Maciej Draguła
Maciej Kaczkowski
Maria Wyrzykowska
Mateusz Borowski
Mikołaj Piórczyński
Paulina Kaczyńska
Piotr Hondra
Piotr Kitłowski
Weronika Piotrowska
Zuzanna Glinka